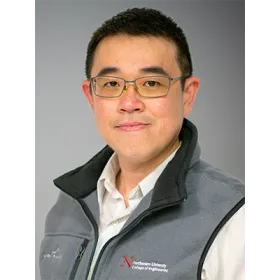
Recent research in safety control has leveraged the availability of accurate models to detect impending safety violations and to intervene accordingly. However, there is often a mismatch between the models that are used for algorithm design and the real systems. Moreover, control designs typically assume the availability of full state information that is error-free and trustworthy. These modeling discrepancies, sensing/estimation errors and the possibility of compromised/spoofed signals, if not proactively considered, will jeopardize safety guarantees, leading to serious damage to safety-critical systems, including autonomous vehicles and power systems, and to loss of trust in these technologies. This talk presents some of our contributions to the development of set-based estimation, control, and learning methods for safe and secure cyber-physical systems under uncertainty.
The first part of the talk will focus on the design of interval observers for estimating the states of various uncertain system classes by leveraging mixed-monotonicity theory, as well as a recent extension to resilient state estimation against false data injection attacks. Next, the talk will discuss tools for computing controlled invariant/recurrent sets with applications to safety control via robust control barrier functions and intent-aware set-based motion planning, control, and estimation. Finally, the talk will conclude with a description of set-membership learning approaches for learning robust inclusion models from noisy data that can be used for robust data-driven safety and (intent) model estimation.