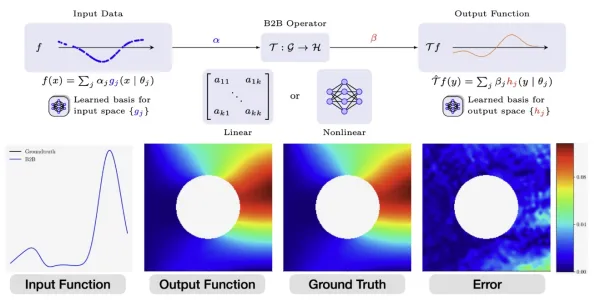
Basis-to-basis operator learning using function encoders
Operator learning is a new frontier in computational learning-based approaches for autonomy that enables us to work directly with function-to-function mappings. We present Basis-to-Basis (B2B) operator learning, a novel approach for learning operators on Hilbert spaces of functions based on the foundational ideas of function encoders.
-Autonomous Systems Group
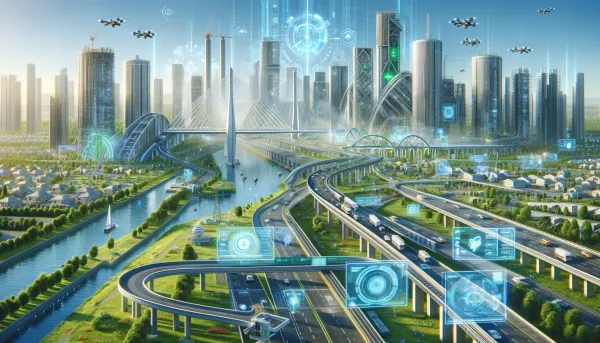
Chishiki.ai: An integrated community of Computational Infrastructure professionals (CIPs) across AI and CEE sectors
Chishiki.ai is an integrated community of CI professionals (CIPs) across artificial intelligence (AI) and civil and environmental engineering (CEE) practices to bolster U.S. infrastructure, aligning with thrust areas identified by the 2020 National Artificial Intelligence Initiative Act and the 2022 Infrastructure Investment and Jobs Act. The Chishiki project adopts four strategies to build a sustainable, diverse, and integrated community of CI professionals for AI in CEE by (1) fostering collaboration between CIPs and domain experts through initiatives such as research summits, graduate and undergraduate fellowships, joint research initiatives, and industrial partnerships; (2) offering personalized and scalable learning environments powered by AI; (3) developing innovative AI-enabled CI architectures for reproducible and efficient workflows; and (4) creating a diverse, sustainable CIP community through engagement with historically underrepresented institutions through recruitment and research initiatives. Chishiki offers AI-enhanced CI solutions and supports an integrated and diverse CIP community dedicated to transforming Civil and Environmental Engineering.
-Geoelements Research Group
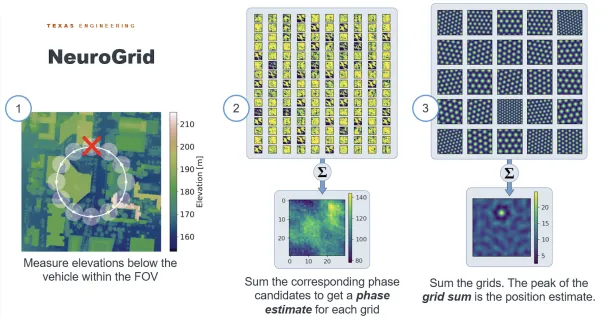
Terrain-Relative Navigation with Neuro-Inspired Elevation Encoding
In traditional approaches to terrain-relative navigation (TRN), terrain measurements are compared to an elevation map carried onboard. These methods are computationally expensive, and it is impractical to store high-quality maps of large swaths of terrain. In this work, we generate position measurements using a novel method inspired by navigation in animals. We incorporate the approach into an inertial navigation scheme using a novel measurement rejection strategy and online covariance computation. Our results show that the filter provides accurate state information over the course of a long trajectory.
- Nonlinear Estimation and Autonomy Research Group

Robust Information Sharing for a Decentralized Team of UAVs in Systems Toolkit (STK)
We extend our state of the art information sharing algorithm to a high-fidelity simulation with advanced sensor and communication models. We ensure that a team of agents performing routine surveillance can self-police and identify if any in their midst are venturing off their nominal flight-paths. We demonstrate three different capabilities for maintaining robust information sharing: (1) resilience against a faulty sensor; (2) reliability in a communication-denied environment; and (3) performance against adversarial hacking.
- Autonomous Systems Group
An Investigation of Time Reversal Symmetry in Reinforcement Learning
One of the fundamental challenges associated with reinforcement learning (RL) in robotics is that collecting sufficient data can be both time-consuming and expensive. In this paper, we formalize a concept of time reversal symmetry in Markov decision processes (MDPs) which builds upon the established structure of dynamically reversible Markov chains (DRMCs) and time reversibility in classical physics. Using this new construct, we investigate the utility of this concept in reducing the sample complexity of robot learning. We observe that utilizing the structure of time reversal in an MDP allows every environment transition experienced by an agent to be transformed into a feasible reverse-time transition, effectively doubling the number of experiences in the environment. To test the usefulness of this newly synthesized data, we introduce a novel approach called time symmetric data augmentation (TSDA) and investigate its application in both proprioceptive and pixel-based state within the realm of off-policy, model-free RL. Empirical evaluations showcase how these synthetic transitions can enhance the sample efficiency of RL agents in time reversible scenarios without friction or contact. We also test this method in less idealized environments, where TSDA can significantly degrade sample efficiency and policy performance, but can also improve sample efficiency under the right conditions. Ultimately we conclude that time symmetry shows promise in robot learning, but only if the environment and reward structure are of an appropriate form.
- Control and Learning for Autonomous Robotics Group
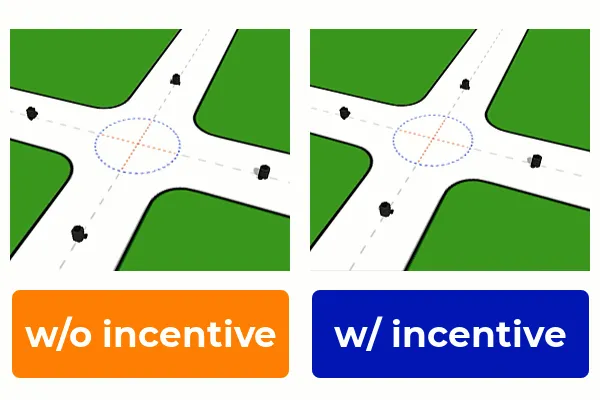
Algorithmic Inverse Games: Inferring What Games You Are Playing
Inverse games are about inferring players’ motives from their interaction history. What motivates a basketball player to make tough shots when double-teamed, or a poker player to call a bluff? Inverse games answer these questions by explaining the players’ past decisions and help predict the players’ future actions.
- Autonomous Systems Group
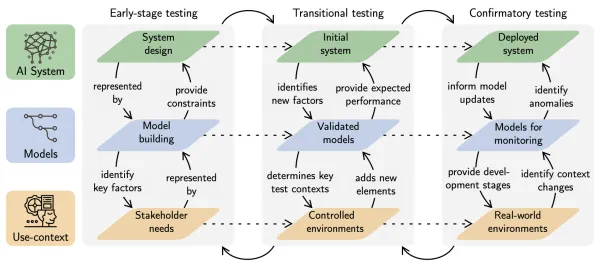
Autonomy Verification & Validation Roadmap and Vision 2045
Researchers at the Center for Autonomy coauthored a NASA report on the vision for autonomy verification and validation. The report brings attention to verification challenges facing the design and deployment of autonomous aircraft in the emerging ecosystem of advanced air mobility. In addition, it proposes a future trajectory for solving these verification challenges as a multidisciplinary research community and conjectures on the enabled technologies that overcoming those challenges might give rise to. A particularly interesting development involves new algorithms and techniques that use learning to train on data posing unique challenges and constitute promising research trajectories within verifying and validating autonomy. Autonomous systems are also increasingly deployed alongside human agents, raising safety, assurance, and regulatory challenges. The report is joint work with industry (Boeing, Collins, and GE), government (NASA), and academia (UT Austin, MIT, and the University of Michigan), thereby developing a comprehensive understanding of these issues.
- Autonomous Systems Group
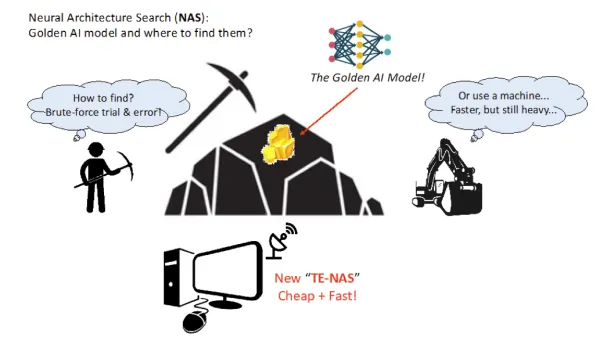
Accelerating Neural Architecture Search with Theory-Grounded, Training-Free Metrics
Over the past decade, the world has seen tremendous increases in the deployment of artificial intelligence (AI) technology. The main horsepower behind the success of AI systems is provided by deep learning models and machine learning (ML) algorithms. Recently, a new AI paradigm has emerged: Automated Machine Learning (AutoML) including its subfield Neural Architecture Search (NAS).
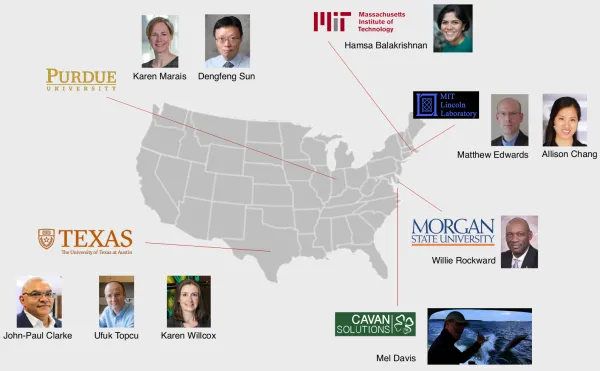
Autonomous Aerial Cargo Operations at Scale
The project addresses the challenge of "safe and efficient growth in global operations, with the expected outcome of an algorithmic foundation that will help realize increasingly autonomous and collaborative air traffic management for all classes of airspace and vehicles and support scalable and efficient operations that rapidly adapt to meet changing demands and to respond to system disruptions.
- Autonomous Systems Group
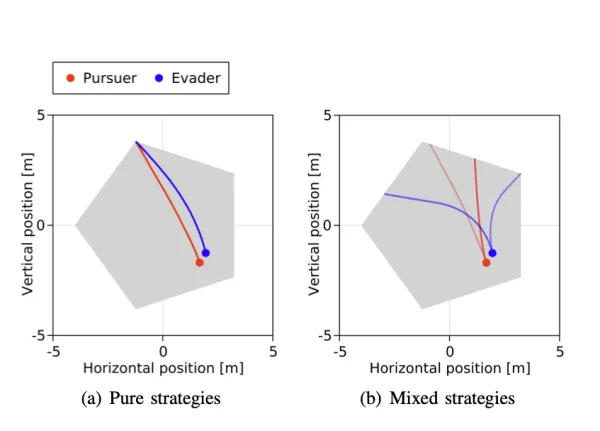
Learning Mixed Strategies in Trajectory Games
In multi-agent settings, game theory is a natural framework for describing the strategic interactions of agents whose objectives depend upon one another’s behavior. Trajectory games capture these complex effects by design. In competitive settings, this makes them a more faithful interaction model than traditional “predict then plan” approaches. However, current game-theoretic planning methods have important limitations. In this work, we propose two main contributions. First, we introduce an offline training phase which reduces the online computational burden of solving trajectory games. Second, we formulate a lifted game which allows players to optimize multiple candidate trajectories in unison and thereby construct more competitive “mixed” strategies. We validate our approach on a number of experiments using the pursuit-evasion game “tag.”
- Control and Learning for Autonomous Robotics Group
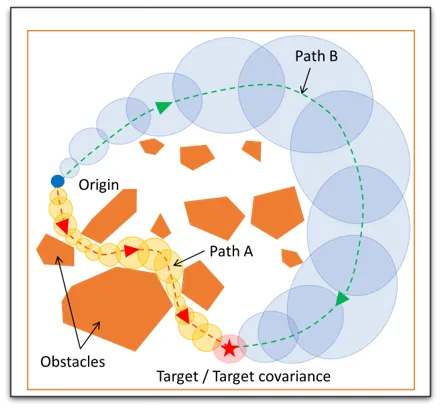
Path Planning for Minimum Sensing Navigation
In this article, we propose a path planning method- ology for a mobile robot navigating through an obstacle-filled environment to generate a reference path that is traceable with moderate sensing efforts. The desired reference path is characterized as the shortest path in an obstacle-filled Gaussian belief manifold equipped with a novel information-geometric distance function. The distance function we introduce is shown to be an asymmetric quasi-pseudometric and can be interpreted as the minimum information gain required to steer the Gaussian belief. An RRT*-based numerical solution algorithm is presented to solve the formulated shortest-path problem. To gain insight into the asymptotic optimality of the proposed algorithm, we show that the considered path length function is continuous with respect to the topology of total variation. Simulation results demonstrate that the proposed method is effective in various robot navigation scenarios to reduce sensing costs, such as the required frequency of sensor measurements and the number of sensors that must be operated simultaneously.
- Networked Control Systems Lab
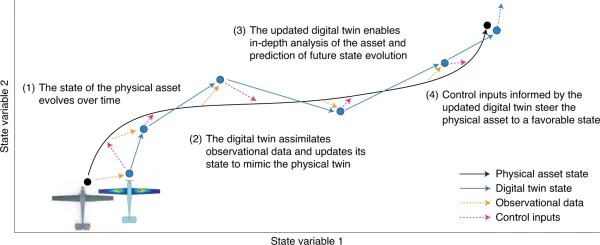
Predictive Digital Twins at Scale for Space Systems
A unifying mathematical formulation is needed to move from one-off digital twins built through custom implementations to robust digital twin implementations at scale. This work proposes a probabilistic graphical model as a formal mathematical representation of a digital twin and its associated physical asset. We create an abstraction of the asset–twin system as a set of coupled dynamical systems, evolving over time through their respective state spaces and interacting via observed data and control inputs. The formal definition of this coupled system as a probabilistic graphical model enables us to draw upon well-established theory and methods from Bayesian statistics, dynamical systems and control theory. The declarative and general nature of the proposed digital twin model make it rigorous yet flexible, enabling its application at scale in a diverse range of application areas. We demonstrate how the model is instantiated to enable a structural digital twin of an unmanned aerial vehicle (UAV). The digital twin is calibrated using experimental data from a physical UAV asset. Its use in dynamic decision-making is then illustrated in a synthetic example where the UAV undergoes an in-flight damage event and the digital twin is dynamically updated using sensor data. The graphical model foundation ensures that the digital twin calibration and updating process is principled, unified and able to scale to an entire fleet of digital twins.
- Nonlinear Estimation and Autonomy Research Group
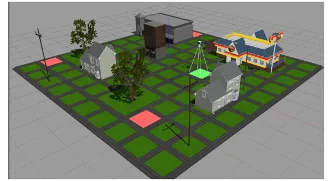
Planning in Adversarial Environments
In any competition, a key to a human team’s success is to be unpredictable. If a football team were to follow the same strategy to score a touchdown in every game, their competitors would exploit this information for their own advantage in the future. Can autonomous systems, like humans, act unpredictably to compete with their adversaries? Is there a limit on the unpredictability of their goal-directed behavior? Combining ideas from stochastic control and information theory, we establish the theoretical limits and computational requirements for unpredictable planning in adversarial environments. We also utilize these insights to develop efficient algorithms that enable autonomous systems to securely carry out tasks, e.g., surveillance, in the presence of adversaries.
- Autonomous Systems Group
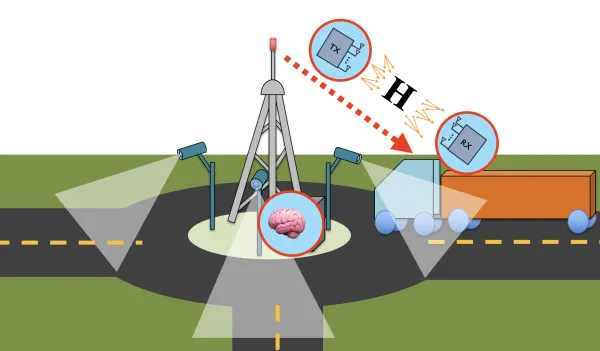
Control System Over Resource-Constrained Network
Our research focuses enabling reliable control over constrained communication networks. Communication is a critical resource when it comes to multi agent autonomy. In many settings, such as when the agents are mobile, the communication will necessarily be wireless. Communication between agents and controllers must satisfy constraints on reliability and latency to ensure both safety and satisfactory performance. Meanwhile, physical wireless resources (like time, bandwidth, and power) are fundamentally scarce. This motivates our research into (1) the design of control systems that use communication resources efficiently and (2) approaches to control-communication co-design that enable reliable control over resource constrained networks.
- Networked Control Systems Lab
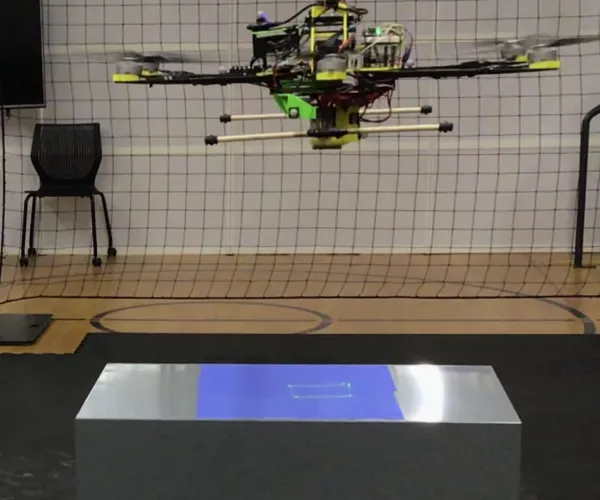
A 3D Printing Hexacopter: Design and Demonstration
3D printing of medium- to large-scale objects is often limited by the size of the 3D printer - the bigger the desired object, the bigger the 3D printer has to be. To address these limitations, we develop a 3D printing hexacopter that uses fused deposition modeling to deposit polymer materials during flight. Recently, we have demonstrated the feasibility of this approach by successfully printing contours of polylactic acid on a flat surface. Future research focuses on developing advanced control algorithms to improve the 3D printing accuracy.
- Autonomous Systems Group

Neuromorphic Vision Sensors
Neuromorphic (event-based) vision sensors are expected to advance the frontiers of high-speed, high-dynamic-range and low-power vision-based robotics in the upcoming years. Event cameras are becoming more and more affordable, and are now widely available in robotics labs where their utility has been confirmed via experiments. In spite of these empirical successes, skepticism persists regarding the superiority of event cameras to conventional frame-based cameras in light of the concurrent developments of low-cost, high-performance CMOS technologies. We propose an information-theoretic comparison of event-based and frame-based vision sampling schemes, which we believe will formally prove (or disprove) the advantages of different schemes.
- Networked Control Systems Lab
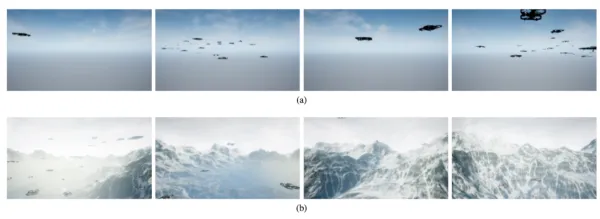
Learning Decentralized Controllers with Graph Neural Networks
A recent paper by members of the DCIST alliance develops a perception-action-communication loop framework using Vision-based Graph Aggregation and Inference (VGAI). This multi-agent decentralized learning-to-control framework maps raw visual observations to agent actions, aided by local communication among neighboring agents. The framework is implemented by a cascade of a convolutional and a graph neural network (CNN / GNN), addressing agent-level visual perception and feature learning, as well as swarm-level communication, local information aggregation and agent action inference, respectively. By jointly training the CNN and GNN, image features and communication messages are learned in conjunction to better address the specific task. The researchers use imitation learning to train the VGAI controller in an offline phase, relying on a centralized expert controller. This results in a learned VGAI controller that can be deployed in a distributed manner for online execution. Additionally, the controller exhibits good scaling properties, with training in smaller teams and application in larger teams. Through a multiagent flocking application, the researchers demonstrate that VGAI yields performance comparable to or better than other decentralized controllers, using only the visual input modality (even with visibility degradation) and without accessing precise location or motion state information.
- Visual Informatics Group