Past Events
Event Status
Scheduled
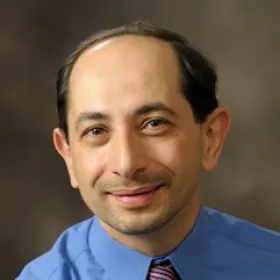
May 14, noon to 1 p.m.
In this presentation we explore the past, present and future potential of AI in Geotechnical Engineering. The presentation will provide a brief history and context of AI and its uses in Civil Engineering in general and Geotechnical Engineering in particular. The presentation will then track developments over the past three decades including the use of Artificial Neural Networks for developing correlations in lieu of conventional regression analysis, as well as the use of ANN to represent material constitutive behavior. The presentation will also explore the role of AI in inverse analysis and discovering material behavior. Lastly the presentation will delve into the world of Large Language Models and Generative AI application in Geotechnical Engineering with several illustrative examples.
Event Status
Scheduled
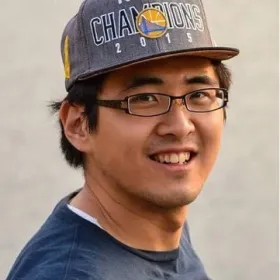
Sept. 13, 2024, 10 to 11 a.m.
POB 4.304
Natural language provides an intuitive and flexible way for humans to communicate with robots. Grounding language commands to structured task specifications enables autonomous robots to understand a broad range of natural language and solve long-horizon tasks with safety guarantees. Linear temporal logic (LTL) provides unambiguous semantics for language grounding, and its compositionality can induce skill transfer.
Event Status
Scheduled
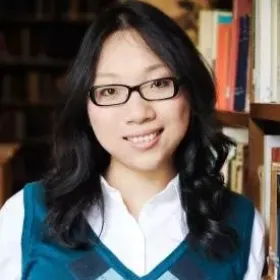
Sept. 11, 2024, 11 a.m. to noon
POB 4.304
Autonomous systems are rapidly transforming various sectors such as transportation and manufacturing by offering innovative solutions that enhance quality of life. However, these advancements bring significant challenges related to safety and trustworthiness. In this talk, I will present my group’s research on addressing some of these challenges. I will start by introducing our approach to planning in autonomous systems, which considers human trust in automation by employing a partially observable Markov decision process (POMDP) to model human-autonomy interactions. I will then highlight our recent developments in safe POMDP online planning, which provide probabilistic safety assurances in dynamic environments. Lastly, I will delve into our work on multi-agent systems, showcasing our efforts to ensure safety and explainability in multi-agent reinforcement learning.
Event Status
Scheduled
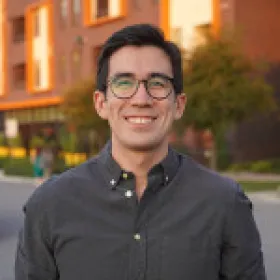
July 17, 2024, 3 to 5:30 p.m.
POB 6.304
How can we transform artificial intelligence (AI) capabilities into engineering systems? That is, how can we engineer AI systems within budget constraints, certify them with respect to stakeholder requirements, and ensure that they meet the needs of the end user? Towards answering these questions, this dissertation develops engineering methodologies for the design of scalable and reliable AI systems, as well as AI algorithms that leverage the unique characteristics of specific engineering problems.
Event Status
Scheduled
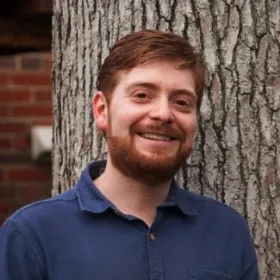
May 13, 2024, 11 a.m. to 12:30 p.m.
POB 6.304
Uncertainty is ubiquitous in virtually all real-world interactions between agents. Without knowing the state of the world or the intentions of others, rational agents must use the limited information at their disposal for decision-making, maximizing their expected payoff and controlling risk. In this setting, information itself becomes valuable: statistical decision theory (SDT) defines the value of information (VoI) as the expected gains from informed decision-making. When a decision-maker has the opportunity to first gather additional information at some cost, VoI provides a principled basis by which to make that decision. We introduce VoI in the multi-agent setting, within games of incomplete information. We use VoI to define a general information design problem, in which an agent has the opportunity to decide how to preemptively gather information, prior to a non-cooperative scenario. We introduce two approaches for solving this problem: a Bayesian optimal, decision-theoretic approach, and an information-theoretic approach based on hypothesis clustering. We demonstrate the decision-theoretic method in a smooth, Blotto-like tower defense game. The clustering approach is demonstrated in sensing allocation for a traffic routing control problem, against exogenous data-poisoning attacks.
Event Status
Scheduled
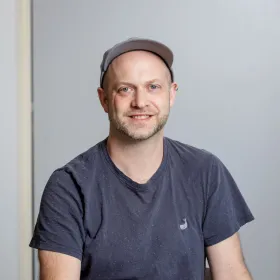
March 26, 2024, 3:30 to 5 p.m.
POB 6.304
In this seminar, we discuss various aspects of artificial intelligence (AI) planning. Our mission is to devise a plan for a robust, resilient, and provably correct autonomous system under real-world conditions. For example, reinforcement learning promises that autonomous systems can learn to operate in unfamiliar environments with minimal human intervention. However, why haven't most autonomous systems implemented reinforcement learning yet? The answer is simple: there are significant unsolved challenges. One of the most important ones is obvious: Autonomous systems operate in unfamiliar, unknown environments. This lack of knowledge is called uncertainty. To tackle these challenges, we combine the areas of AI and Formal Methods and employ neurosymbolic AI methods to achieve trustworthy, reliable, and safe artificial intelligence
Event Status
Scheduled
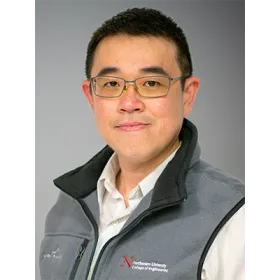
May 8, 2023, 11 a.m. to noon
POB 6.304
Dr. Sze Zheng Yong is an Associate Professor with the Department of Mechanical and Industrial Engineering, Northeastern University, Boston, MA, USA. Prior to that, he was an Assistant Professor in the School for Engineering of Matter, Transport and Energy at Arizona State University and a postdoctoral fellow in the Department of Electrical Engineering and Computer Science at the University of Michigan, Ann Arbor. He received a Dipl.-Ing. (FH) degree in Automotive Engineering with a specialization in mechatronics and control systems from the Esslingen University of Applied Sciences, Germany in 2008, and S.M. and Ph.D. degrees in Mechanical Engineering from Massachusetts Institute of Technology, Cambridge, MA, in 2010 and 2016, respectively. Dr. Yong was the recipient of the DARPA Young Faculty Award in 2018, the NSF CAREER and NASA Early Career Faculty awards in 2020, and the ONR Young Investigator Program Award in 2022. His research interests include the broad areas of control, estimation, planning, identification, and optimization of hybrid systems, with applications to autonomous, robotic, and cyber-physical dynamic systems and their safety, robustness, and resilience.
Event Status
Scheduled
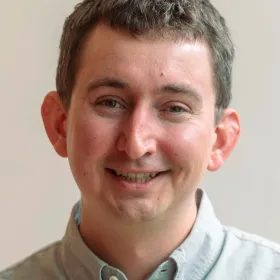
May 3, 2023, 3 to 4:30 p.m.
Daniel Fried is an assistant professor in the Language Technologies Institute at Carnegie Mellon University since Fall 2022. His research in natural language processing focuses on grounding, interaction, and applied pragmatics, with a particular focus on language interfaces such as grounded instruction following and code generation. Previously, he was a postdoc at Meta AI and the University of Washington and completed a PhD at UC Berkeley. His work has been supported by a Google PhD Fellowship and a Churchill Fellowship.
Event Status
Scheduled
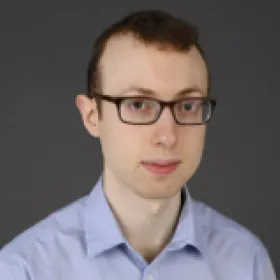
April 10, 2023, 11 a.m. to noon
POB 6.304
Jared Miller is a 5th year PhD Student at the Robust Systems Lab at Northeastern University, advised by Mario Sznaier. He received his B.S. and M.S. degrees in Electrical Engineering from Northeastern University in 2018. He is a recipient of the 2020 Chateaubriand Fellowship from the Office for Science Technology of the Embassy of France in the United States. He was given an Outstanding Student Paper award at the IEEE Conference on Decision and Control in 2021 and in 2022. His current research topics include safety verification and data-driven control. His interests include large-scale convex optimization, nonlinear systems, semi-algebraic geometry, and measure theory.
Event Status
Scheduled
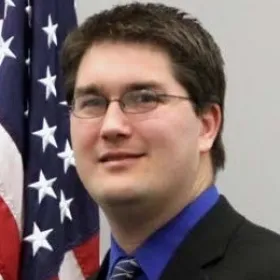
March 24, 2023, 1:30 to 2:30 p.m.
POB 6.304
Dr. Jared Culbertson is a research mathematician with the U.S. Air Force Research Laboratory's Autonomous Capabilities Team (ACT3), a research group focused on the development and deployment of flexible AI solutions across a diverse set of air and space mission areas. Jared's research primarily deals with fundamental aspects of representational structures, recently involving compositional approaches for hybrid dynamical systems and now focused on behavior acquisition, diversity, and composition in reinforcement learning problems.